Imagine relying on a machine to make decisions that could shape your career or safeguard your health. As AI technology continues to advance, we stand at the crossroads of a new era where life itself will become entwined with AI. We face a near-term future where we must strike a delicate balance of how and when to use AI. Two pivotal elements bridge the gap to full AI adoption in the future: trust and experience.
Unlike humans, who learn from the ups and downs of life, AI systems are trained on vast datasets without ever experiencing the world firsthand. They don't understand trust because they've never been betrayed or rewarded by it. This fundamental difference prompts an important question: how can we place our trust in machines that lack the intrinsic experiences that shape human trust? As AI becomes more integrated into our lives, grappling with this dilemma will be essential for our future success.
The Trust Gap
Trust is crucial for the adoption of AI in business and personal applications. Today, Generative AI is often used for low-stakes tasks, such as writing an email or creating a meme to send to a group chat—situations where outcomes don’t significantly change or even matter. On the other hand, more established types of AI and machine learning have become widely used in very high-stakes tasks such as in healthcare, where AI-based tools are supplementing doctors for both diagnostics and surgical procedures. For these types of tasks, the tools undergo rigorous vetting processes, ensuring they supplement or exceed the performance of humans operating without them.
The real challenge of trust with AI lies in the middle ground—tasks that impact daily lives or careers but are not life-threatening. This middle ground raises the question: when can we trust AI to perform tasks that, if done wrong, could have serious but not life-threatening consequences? These scenarios have not been thoroughly vetted or regulated like healthcare or self-driving but could have catastrophic individual consequences if problems arise, from being fired to losing massive amounts of money.
Consider the concept of the horseshoe theory, where polar opposites often converge.
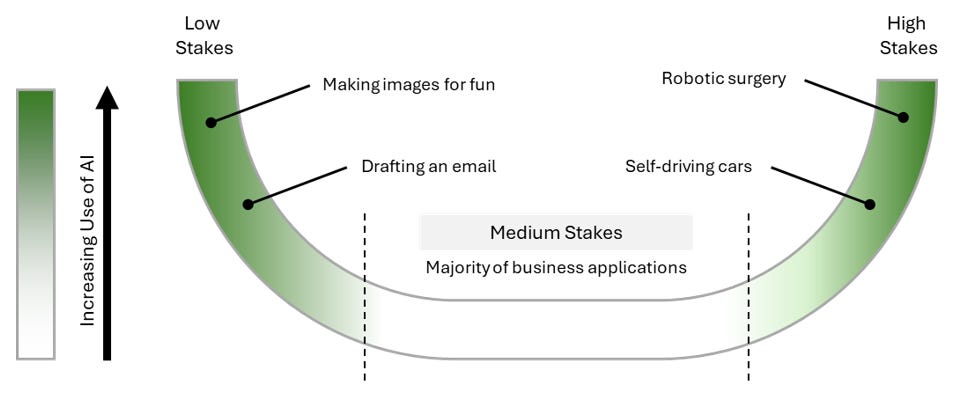
The easiest use cases for AI adoption are those that are either simple and low risk, where GenAI has started to gain traction, or so high risk that they require extensive vetting to ensure safety. Many applications of AI started from one or the other of these extremes, but soon we’ll be presented with challenges in the middle of this spectrum—significant but not life-defining significant.
Adoption in Business
In the business world, trust is paramount. Companies are constantly looking to increase their efficiency, which drives the adoption of software tools. Integrating any software, especially AI tools, into business processes requires confidence in its reliability. Point solutions, where AI handles specific tasks under human supervision, are already widely accepted. At this point, no one bats an eye at basic customer support chatbots or machine learning-based algorithms that help with scoring sales prospects.
However, as the scale and scope of AI's responsibilities grow and GenAI becomes more widely used, the challenge of trust becomes more pronounced. Trust may reach a tipping point with the advent of autonomous AI agents capable of acting independently. These agents promise increased productivity by fully carrying out tasks on their own. They can comprehend goals, devise tasks to achieve those goals, execute the tasks, adjust their priorities, and learn from their experiences to reach desired outcomes. This autonomy allows AI agents to perform massive amounts of work without human oversight, potentially delivering substantial cost savings and revenue gains to businesses.
Take the example of AI sales agents. Companies like Salesforce and HubSpot are pushing hard to develop sales-adjacent AI capabilities. It’s not far-fetched to envision AI agents that could perform outreach, log calls, note follow-ups, and coordinate with stakeholders in the near future. But would we trust them?
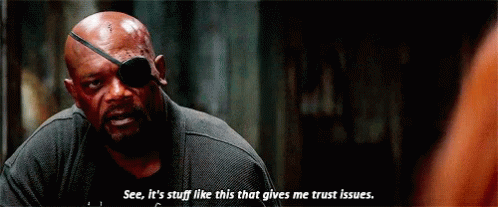
Larger enterprises tend to adopt a cautious approach due to economic and reputational risks, while smaller businesses may leverage their agility to experiment more freely with AI tools. Businesses will need to calculate the cost-benefit tradeoffs of adoption as these tools come to market. This decision-making process will likely evolve into a greater exercise in game theory, where businesses don’t want to be too early or too late in adopting AI. So, what’s the tipping point? Trust. Both organizational trust and societal trust in these systems to succeed and deliver results without compromising what else businesses care about.
How Do We Know What to Trust?
Unfortunately, AI models are far too complex for most individuals to fully understand or verify, and many aspects of them remain a black box even to AI researchers. Leading AI experts and early adopters can help by vetting solutions and providing word-of-mouth testimonials. However, with the potential for a single error to cause significant harm, businesses will be cautious about their rollouts and will look for external validation from experts. But in the grand scheme of things, is the ability to trust an AI to complete a task that different from our trust in a human?
Human trust stems from real-world experiences and learnings. We’ve come to accept as facts what authorities in their fields and textbooks have told us. We trust the people around us, often without being able to explain why. Much of it may be cultural or innately ingrained in our brains, making it difficult to articulate. So why should trusting AI agents be any different from trusting other humans?
In a work setting, successful businesses will outline KPIs for the success of their AI initiatives and use those metrics to guide broader implementation. AI agents pose such a significant risk of disruption that businesses will be compelled to pilot these programs or risk falling behind. Smart businesses will adopt AI in sandbox environments, pushing for steady rollouts to gradually increase efficiency without taking outsized risks.
Start Small with Pilot Programs: Implement AI systems in controlled, low-risk environments to test their effectiveness before wider adoption. This allows for performance evaluation and identifying potential issues without significant impact.
Measure Success with KPIs: Establish clear key performance indicators (KPIs) such as accuracy, efficiency, user satisfaction, and cost savings to assess the AI's performance and make data-driven decisions on scaling its use.
Gradual Rollout: Gradually expand AI’s responsibilities based on performance metrics. Start with automating repetitive tasks and progressively move to more complex tasks as confidence in the AI system grows.
Continuous Monitoring and Feedback: Continuously monitor AI systems and gather feedback from users to ensure they perform as expected and adapt to any changes or new requirements.
The Path Forward: A Symbiotic Relationship
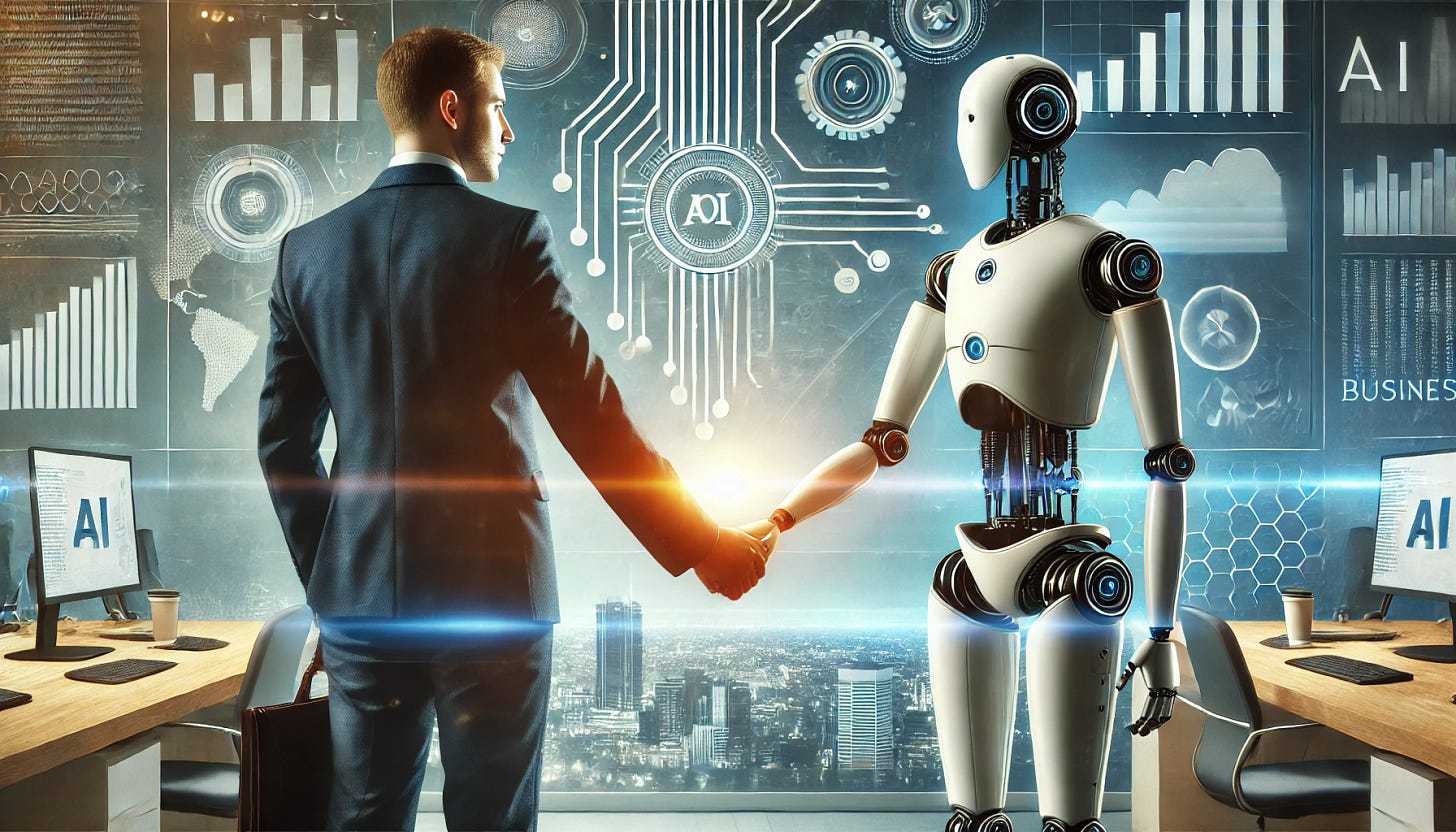
The path forward involves fostering a symbiotic relationship between humans and AI. In time, humans will need to learn how and when to fully trust AI. This trust may develop gradually or all at once as we reach critical tipping points.
Conversely, we may need to make AI easier to trust. What AI truly lacks today is lived experience. If we could improve AI’s ability to gain real-world experience, we can enhance its ability to contextualize data and perform better. Various methods of building AI could provide a proxy for experience—personalized AI, decentralized AI, and advancements in robotics all hold potential. Perhaps we even need to simulate life experiences so AI can develop its own perspective and understanding of the world.
Addressing both the trust and experience gaps is essential for the future of AI. By fostering a symbiotic relationship between humans and AI, we can unlock new possibilities for enhanced productivity and innovation, paving the way for a future where trust and experience are harmoniously integrated into the AI landscape.