The SaaS model has defined a generation of B2B tech startups. It’s brought a steady stream of revenue to companies, scaled seamlessly, and transformed business technology. But in today’s rapidly evolving AI landscape, the foundation of B2B SaaS is shifting. AI accelerates product development, disrupts pricing structures, and creates a complex environment where differentiation is increasingly challenging. The B2B SaaS model itself may not be “dead,” but it is fundamentally changing—and startups must evolve to survive.
To thrive in this AI-driven era, solving a problem is no longer enough. AI’s rapid advancement is eroding traditional moats, leaving companies that lack proprietary data or algorithms especially vulnerable. Startups must develop technically far superior products that stand out in a market crowded with new entrants offering AI solutions and enterprises now able to custom build solutions in-house easier than ever. Equally critical is crafting a go-to-market strategy that not only highlights this technical edge but also demonstrates its undeniable value to users.
The time when a novel SaaS product could carve out a niche simply by solving a problem is coming to an end. With AI tools evolving at breakneck speeds, what once took years to develop can now be achieved in months, weeks, or potentially even days. In this landscape, B2B SaaS companies must rethink their approaches and strategies, and the only way forward is to adapt. So, what exactly is happening to B2B SaaS?
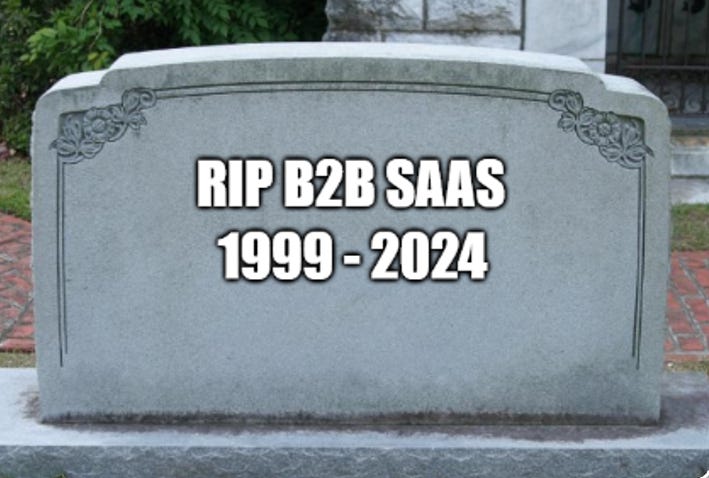
AI Accelerates Development and Deployment
One of the most transformative shifts in today’s tech world is the speed at which AI accelerates product development. Tools like GitHub Copilot and Cursor enable engineers to generate and refine code with unprecedented efficiency, reportedly boosting development speed by over 50%. And these tools continue to evolve—Cursor has integrated AI agents for enhanced collaboration, while Codeium’s new platform, Windsurf, empowers even non-technical users to transform their ideas into functional code by leveraging AI for writing and debugging. The result is a landscape where innovation can move faster than ever before, with barriers to entry for software development dramatically reduced.
While this speed democratizes development, it also creates a significant challenge for startups: speed alone is no longer a differentiator. When anyone can build quickly, rapid iteration becomes a baseline expectation rather than a competitive advantage. Startups must look beyond the race to launch and focus on crafting products with lasting impact. This means prioritizing areas that AI tools cannot easily replicate, such as addressing deeply complex problems, building proprietary technology, or embedding products seamlessly into existing ecosystems. Equally important is recognizing the human factor—leveraging personal connections, strategic distribution, and customer relationships to create a competitive edge that technology alone cannot replace.
AI Shifts the Economics of Build vs. Buy
In the past, the decision to buy or build software was straightforward. Buying was often faster, more reliable, and came with vendor support, while building was seen as time-consuming and resource intensive. But with AI’s rise, this economic calculation is shifting.
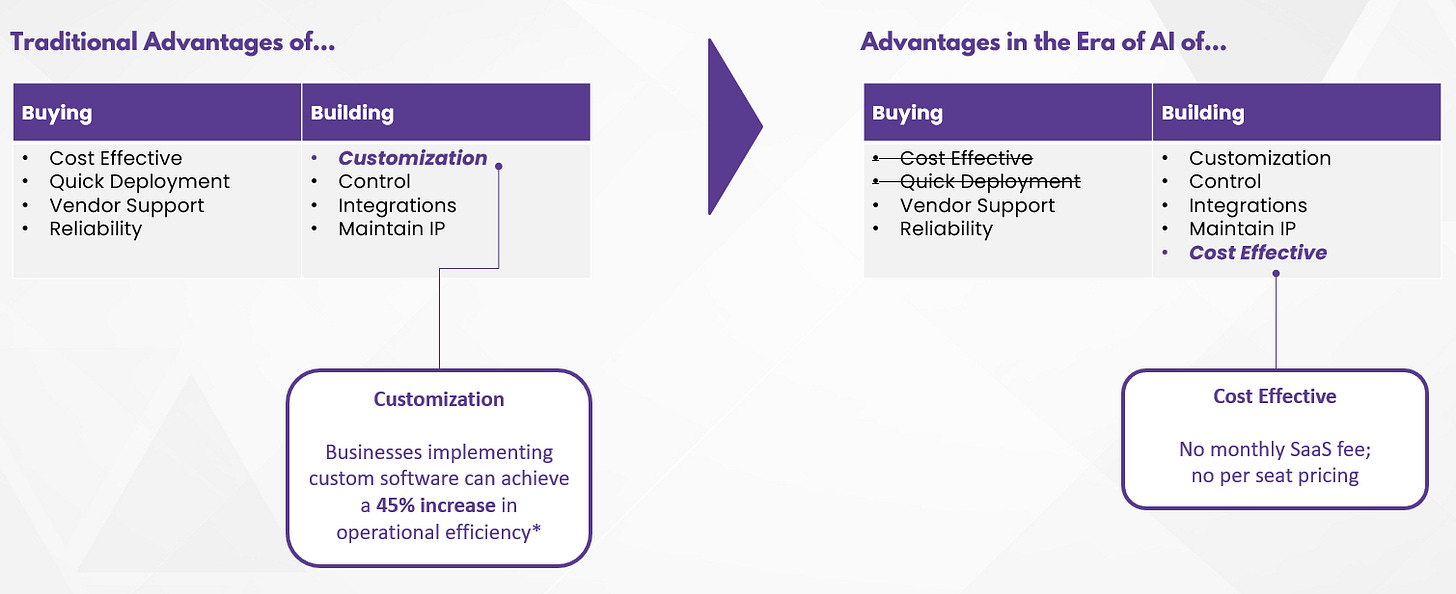
Now, AI-driven tools have made building custom solutions more accessible. Companies like Klarna have made headlines for ripping out traditional SaaS providers like Salesforce, instead opting to build in-house solutions that they can control and customize to fit their exact needs. The reduced reliance on external SaaS providers gives companies more autonomy and control, without the recurring costs associated with licensing fees. Startups must now be cognizant of this shifting landscape where their customers today may be able to replicate a similar solution at a fraction of the cost using AI tools whether in-house or with outsourced developers.
AI Threatens Traditional, Seat-Based Pricing Models
Seat-based pricing has long been a reliable revenue model for B2B SaaS. Companies charge by the user, creating a predictable and scalable revenue stream. But AI is disrupting this model as well. By increasing productivity and efficiency, AI reduces the need for large teams, potentially diminishing the demand for seat-based licenses.
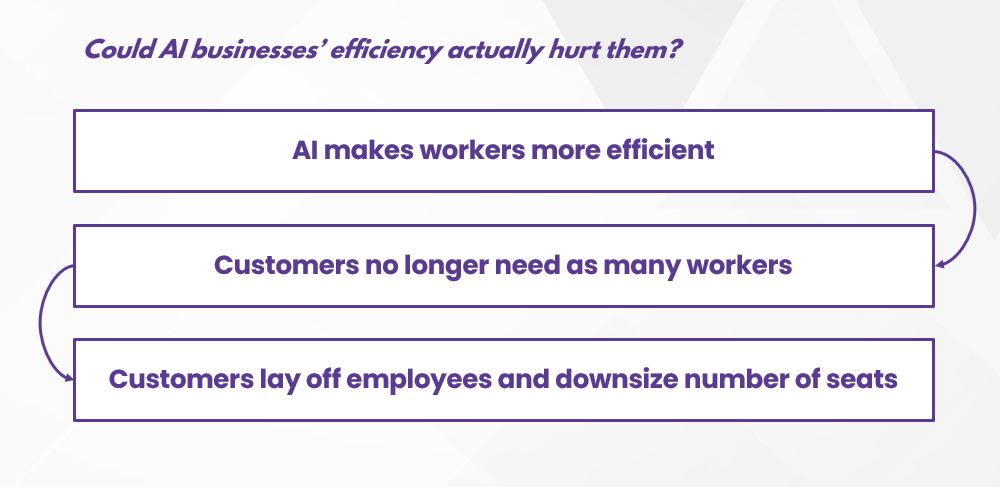
As a result, B2B SaaS companies face a dilemma: fewer users mean fewer seats, which could erode their revenue base. Startups utilizing a seat-based pricing model could risk cannibalizing their own growth by … delivering too much value to their customers. This shift requires founders to think beyond seat-based pricing and consider alternative models that align with the value their product delivers—whether it’s usage-based, performance-based, or something entirely new. The challenge is to adapt pricing in a way that captures AI-driven efficiencies without sacrificing revenue potential.
Perhaps we’ll see a rise in usage-based pricing models, similar to those used by OpenAI and Anthropic, where price is dictated by the number of tokens consumed. For applications geared toward businesses, usage may not translate directly into token counts but could be tied to more tangible business metrics, such as the number of processed transactions, API calls, or workflows automated. This approach ensures customers pay in proportion to their usage while allowing creators to retain healthy margins.
Alternatively, value-based pricing could gain traction, particularly if software leveraging AI can quantify the value it generates for customers. For example:
Revenue Generation: Pricing could be tied to the revenue uplift created by AI-powered recommendation engines in e-commerce platforms.
Cost Savings: Tools that automate manual workflows might charge a percentage of the labor costs saved.
Risk Mitigation: Cybersecurity platforms could justify pricing based on the reduction in potential breach costs.
AI Makes It Even Harder for Customers to Select Software Vendors
B2B procurement processes often begin with customers compiling a checklist of requirements and identifying tools that could meet those needs. From there, they proceed to demos, hoping to determine which option stands out. However, at this stage, customers frequently struggle to differentiate between the available choices. In markets where every product claims to have many of the same features, distinguishing real value becomes a daunting task.
Differentiating based on technical merit alone is rarely viable, especially for buyers without deep technical expertise. Instead, many rely on proxies like review platforms such as Gartner and G2 to guide their decisions. While these platforms provide some structure, they often fail to capture the unique nuances of each product, leaving customers without the transparency they need to make confident choices.
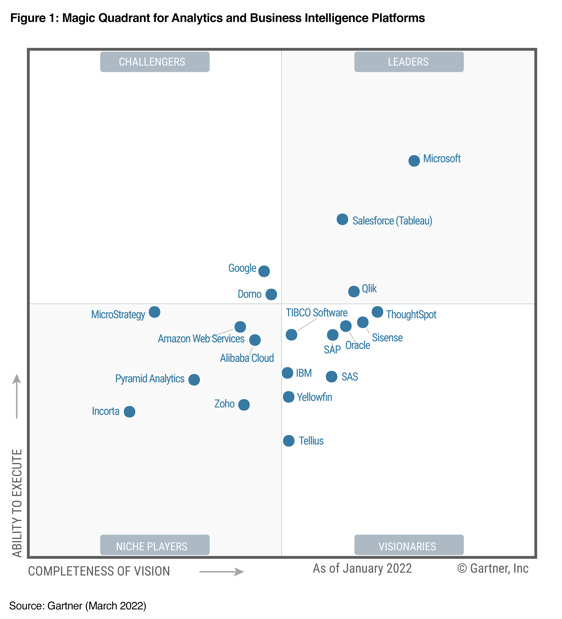
AI complicates this even further. Evaluating the performance of AI models, for instance, is inherently complex and often requires specialized knowledge. Benchmarks like those used for large language models (LLMs) attempt to provide clarity, but they only tell part of the story. Even if a product scores well on an LLM leaderboard, it doesn’t necessarily mean it will deliver the outcomes a business needs. Translating model performance into practical, measurable value within a specific context remains a significant challenge.
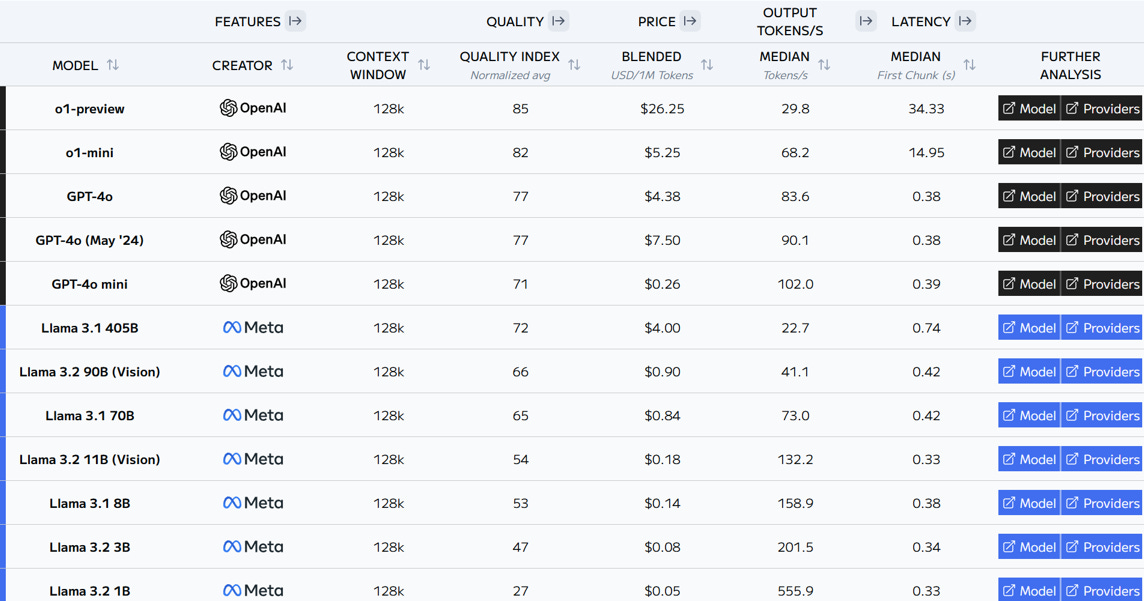
This issue especially extends to the products themselves. AI-driven features are often presented as transformative, but for many customers, it’s difficult to assess how those features translate into real-world impact. Does the product truly solve their unique business problem? Does it integrate seamlessly with their existing workflows? These questions are rarely addressed in a way that feels tangible to buyers.
The Future of B2B SaaS
The B2B SaaS model isn’t disappearing, but it’s facing a transformation. Founders can no longer rely on the same playbook that worked in the past. Instead, they must adapt to AI’s influence on product development, economic dynamics, pricing, and customer expectations.
In this new landscape, the moat is shifting—technical differentiation will only be part of the equation. Success will increasingly depend on stronger distribution strategies and maintaining a human touch (or at least human-appearing) in customer-facing interactions, building genuine connections that resonate beyond automated exchanges.
The search for a moat isn’t easy, but in a world reshaped by AI, it’s more necessary than ever. Stay tuned for my future articles exploring some of the potential ways of creating meaningful differentiation for sustainable B2B software businesses.